Watch out! Vokenization is coming!
Computers are learning the colour of sheeps. Here is why it matters.
A non-negligible part of so-called Artificial Intelligence (AI) consists of making computer really understand what human beings tell or write them in their natural languages, and to answer in kind, so well to be confused with other human beings. That is no easy task.
What is GPT-3, and its limits (until now)
The GPT-3 program is software that emulates understanding of natural language, and is therefore able to engage in general conversations with human beings.
Technically speaking, GPT-3 is a transformer, that is software that “learns to speak” that is to have a “language model”, by being fed huge amounts of written text, and processing them. More in detail, transformers like GPT-3 learn the patterns of human language by observing how words are used in context and then creating a mathematical representation of each word, known as a “word embedding,” based on that context.
The limit of this kind of language models is that they lack common sense, because “looking” at words and sentences, without larger experiences of the world, is not enough to understand it.
One reson why this happens is that the corpuses of text used by language models like GPT-3 are both too literal (literally!) and a bit too removed from reality, to the extent that written text is an intrinsically not-complete representation of reality, includes metaphors, and so on. A language-only model could easily affirm that “real sheeps are all black”, simply because it has encountered many times sentences like “He was the black sheep of the family”.
Here comes vokenization
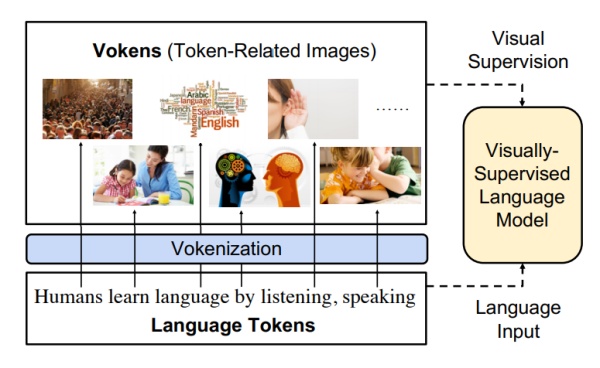
<u><em><strong>CAPTION:</strong>
<a href="https://laptrinhx.com/what-is-vokenization-and-its-significance-for-nlp-applications-3910268356/" target="_blank">Source: LaptrinhX</a>
</em></u>
This was the situation so far. Now, some researchers have developed a new technique that could lead to the next big breakthrough in common sense AI
This new technique, called “vokenization,” should gives language models like GPT-3 the ability to “see.” Here is the idea:
The already existing software programs that recognize images, are “image models” that learn by automatic analysis of photographs. Therefore, while they would not be able to talk about sheeps, they would know (if given the right pictures, of course) that sheeps are almost always white.
This is why having “visual language models”, that is sets of connected images and text that a software could study, would be very useful. Such models could teach AI software like GPT-3 how objects interact with each other, and what real features they have.
Concretely, those visual language models would be huge collection of images with descriptive captions, that is not simply “sheeps” but “sheeps mating in a pen” or “sheeps chased by a wolf, in a meadow”.
The problem is that adding all those captions, to enough images to make a difference, would take forever. The already existing collections of properly captioned images are “simply not enough data to train an AI model for anything useful”.
The news is that researchers have developed this techique, called “Vokenization” that solves this problem automatically, generating visual-language model that work much better than past ones in some of the hardest tests used to evaluate AI language comprehension today.
OK, but what does “vokenization” mean?
The words that are used to train AI language models are known as tokens. By extension, a voken (“visual token”) is the image associated with each token in a visual-language model, a vokenizer is the algorithm that finds vokens for each token, and vokenization is the whole process.
The news is that some researchers have found a way to generate vokens automatically, without anybody having to spend years to manually write captions to describe the images. Details are in the article if you are interested, but this is the gist of it.
What next?
This new technology sensibly improve the already relevant natural language capabilities of programs like GPT-3. This is big, because software that can simultaneously “converse” and “see” is much more useful than software that can only do any one of those things. A talking robot concierge would be worthless if it could not recognize a human waving at it, would it now?
So, what next? “Maybe you can use the same thing to connect the robot’s senses to text." Sommelier robots? Stay tuned.
Who writes this, why, and how to help
I am Marco Fioretti, tech writer and aspiring polymath doing human-digital research and popularization.
I do it because YOUR civil rights and the quality of YOUR life depend every year more on how software is used AROUND you.
To this end, I have already shared more than a million words on this blog, without any paywall or user tracking, and am sharing the next million through a newsletter, also without any paywall.
The more direct support I get, the more I can continue to inform for free parents, teachers, decision makers, and everybody else who should know more stuff like this. You can support me with paid subscriptions to my newsletter, donations via PayPal (mfioretti@nexaima.net) or LiberaPay, or in any of the other ways listed here.THANKS for your support!