When (Big) Data Fails...
unintended consequences explode. Or remain.
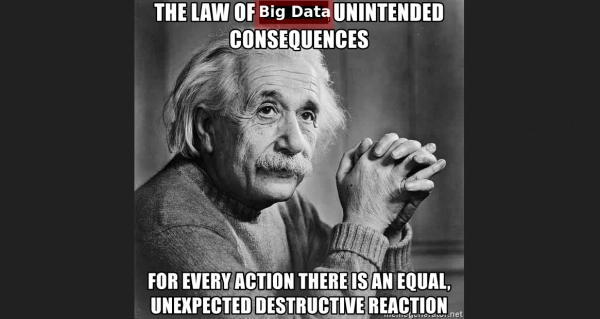
Exactly one year ago, the Journal of Information, Communication and Society published a paper by Anna Lauren Hoffman titled Where fairness fails: data, algorithms, and the limits of antidiscrimination discourse. The paper examines three limits of “antidiscrimination discourses for bringing about the change demanded by social justice”, namely:
- an overemphasis on discrete ‘bad actors'
- single-axis thinking that centers disadvantage
- an inordinate focus on a limited set of goods
This observation in the (very interesting!) paper particularly impressed me:
“When automated decision-making tools are not built to explicitly dismantle structural inequalities, their increased speed and vast scale intensify them dramatically."
Is this still possible?
Maybe (probably) I am missing something, but that sentence seems to sit on the belief that it is possible to build automated tools like that. Really?
Is it still possible to assume, even in theory, that any automated decision-making tools would always, surely “‘explicitly dismantle’ all its designers wanted to explicitly dismantle, and nothing else?
The paper itself warns against, among other things:
- a narrow conception of discrimination contingent on the identification and isolation of discrete perpetrators ‘mechanically linked' to discrete discriminatory outcomes.
- efforts to isolate ‘bad data,' ‘bad algorithms,' or localized biases of designers and engineers [because such efforts are intrinsically] limited in their ability to address broad social and systemic problems.
- single-axis thinking in the law [that] actively produces vulnerabilities for certain groups - specifically Black women - while also over focusing on disadvantage, thus obscuring the production of systematic advantage.
- efforts to design and audit algorithmic systems in the name of ‘fairness' [that] have been hindered by a similar one-dimensional, disadvantage-centered focus.
All these risks are concrete and, so to speak, “issue-independent”. They exist even if the goal is “to explicitly dismantle structural inequalities”. Part of the reason is, of course, that, in order to work well any “automated decision making tools” should receive only “good data”, or fool proof criteria to always recognize “bad data”. And then there is the little problem of explaining, in machine-usable terms, what exactly is structural inequality, and how to “dismantle” it.
The conclusion is always the same
Always collect and use lots of data, without trusting them blindly, as bases for decisions, or to simulate policy outcomes. That, yes. It should be almost always better than a finger in the air.
Never use, or even try to propose, really automated decision making tools to “do justice”, whatever meaning of justice you are talking about.
(This post was drafted in May 2020, but only put online in August, because… my coronavirus reports, of course)
Who writes this, why, and how to help
I am Marco Fioretti, tech writer and aspiring polymath doing human-digital research and popularization.
I do it because YOUR civil rights and the quality of YOUR life depend every year more on how software is used AROUND you.
To this end, I have already shared more than a million words on this blog, without any paywall or user tracking, and am sharing the next million through a newsletter, also without any paywall.
The more direct support I get, the more I can continue to inform for free parents, teachers, decision makers, and everybody else who should know more stuff like this. You can support me with paid subscriptions to my newsletter, donations via PayPal (mfioretti@nexaima.net) or LiberaPay, or in any of the other ways listed here.THANKS for your support!