What we already knew about (big) data-driven inequality
Warnings and examples of algorithms and “Big Data” that exploit or discriminate people in ways unknown to their very authors appear more and more frequently in the news. But they are not news.
Here are just a few quotes to prove that (continuing to) “move fast and break things” is not a good idea.
Data-Driven Discrimination Warnings
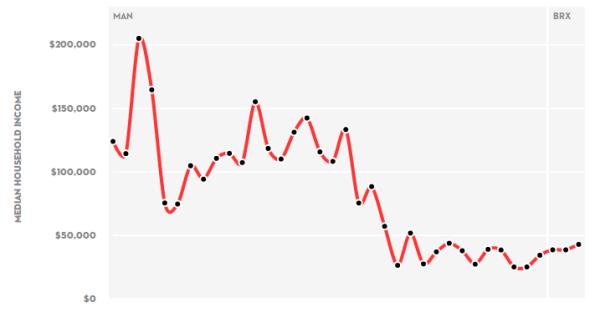
Median Household income inequality on one New York subway line
</em></u>
The graph above, coming from here, is agreat example of how analysis of “big data” greatly facilitates the visualization and comprehension of inequality. All too often, however, big data are used to hide or preserve inequality. Consider these cases:
1: Redlining is the (many) decades-old discriminatory practice of “refusing or limit loans, mortgages, insurance, etc., within specific geographic areas”. Today, redlining can happen at the hand of well-meaning coders crafting exceedingly complex algorithms that, by directly learning from one another, become inscrutable even to their creators.
2: [Totally] Computerized welfare management reduces caseloads [by] blindly—kicking out recipients whether or not they’re able to get back on their feet. That’s greater inequity, not less, and here is a concrete case of big data helping states kick poor people off welfare
3: User inputs to configure apps and schedules for public transit systems? A good idea, but sufficient only if all users can contribute equally. Problem is, poorer localities often lack the resources to produce or share transit data, meaning some neighborhoods become dead zones.
4: The fact that DNA links you with unknown descendants, ancestors or distant relatives makes it VERY hard to predict how law enforcement or others might use your DNA information in the future.
5: In some cases, discriminatory conditioning happens because of the pervasiveness of collecting and sharing information, making it hard to control who knows what about you. [What if] information about what you’re doing in a particular space gets matched and integrated with existing profiles? [It may be used] to market predatory products to low-income populations or, worse yet, use data to shape rental terms or housing opportunities.
6: More and more data collection, analysis, and use [is] a real threat that things are going to go badly, and disproportionately burden the poorest and most marginalized among us.
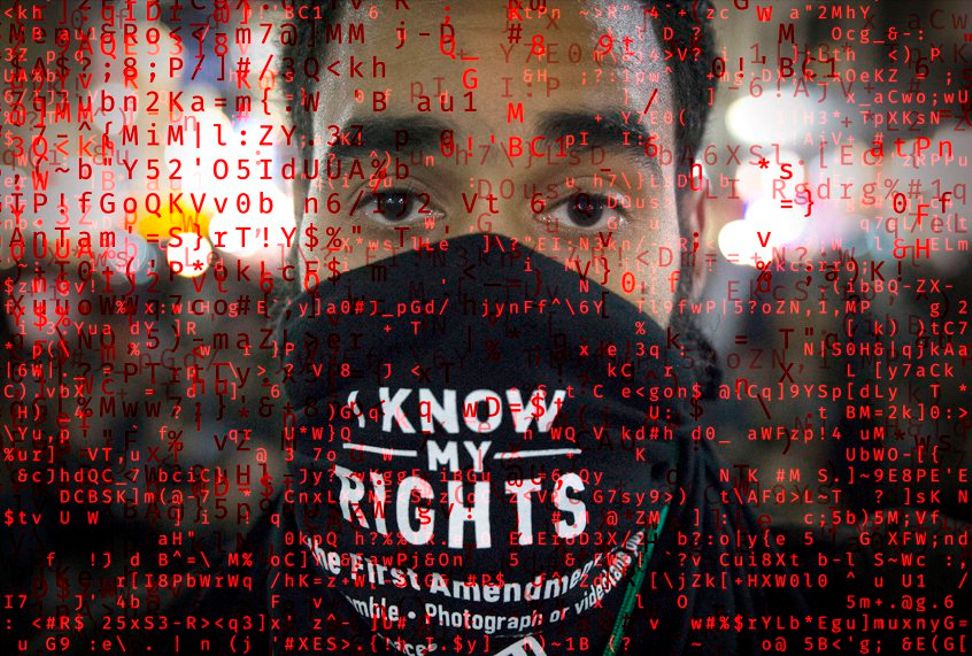
As proof of the last point…
let’s consider the proportion of people living under $7.40/day, which is the minimum necessary to achieve basic nutrition and normal life expectancy.
7: Over the past few decades, that proportion has fallen from 71.8% in 1990 to 58.1% in 2013. But in terms of absolute numbers, a very different story emerges. The number of people living under $7.40/day has grown from 3.78 billion in 1990 to 4.16 billion in 2013.
You cannot make informed decisions if you don’t always look at both numbers together. But above all:
8: the morally relevant metric of progress against poverty is neither absolute numbers nor proportions, but rather the extent of global poverty compared to our capacity to end it. By that metric, we are doing worse than at any time in history. If the poverty rate stays the same while our capacity to end it doubles, then the moral egregiousness of poverty is twice as bad as it used to be.
9: [and it turns out that] Our capacity to end poverty has improved by a factor of 3.31 [since 1990]. By this method, the moral egregiousness of poverty is 2.69 times worse than it was in 1990.
We already knew this BEFORE it became big “news”
The first 6 points above are almost literal quotes from a 2014 article, before the opacity of Facebook (or any other similar platform, of course), its impact on political elections or ineffective schemes to break it became daily news. The last three quotes show how easy it can be to misrepresent inequality via big data, are from here. You should compare that example with the signals that worldwide development aids may be misdirected because “Everything we have heard about global urbanization turns out to be wrong”. Much more of the same is available in:
- “Algorithms reproducing social and racial inequality” (source of the image above)
- “Automating inequality”
- Weapons of Math Distruction".
We need more accountability in the world, and that goal cannot be achieved without more, obviously open data about what is happening. But we cannot ignore anymore the downsides, or leave decisions and policies entirely to technicians. We have already done it, and the result is what, as I tried to show in this post, we already knew almost before starting. “Big Data problems we face today can be traced to the social ordering practices of the 19TH CENTURY”.
Who writes this, why, and how to help
I am Marco Fioretti, tech writer and aspiring polymath doing human-digital research and popularization.
I do it because YOUR civil rights and the quality of YOUR life depend every year more on how software is used AROUND you.
To this end, I have already shared more than a million words on this blog, without any paywall or user tracking, and am sharing the next million through a newsletter, also without any paywall.
The more direct support I get, the more I can continue to inform for free parents, teachers, decision makers, and everybody else who should know more stuff like this. You can support me with paid subscriptions to my newsletter, donations via PayPal (mfioretti@nexaima.net) or LiberaPay, or in any of the other ways listed here.THANKS for your support!